What is a QR Code?
Invented by a Japanese company in 1994 to label automobile parts, Quick Response codes, best known as QR codes, are rapidly becoming ubiquitous everywhere in the world. Their design, inspired by the board and black and white pieces of the game of Go, permits the storage of more information than regular barcodes and to access that information more quickly. The COVID-19 pandemic contributed to their increased popularity as it conveniently replaced physical media of all types for the purpose of content sharing. It is now common to see them in restaurant menus, plane tickets, advertisements and even in stickers containing minimal to no text pasted on lamp posts and other surfaces, enticing passers-by to scan its content.
QR Code Phishing Attacks (Quishing)
Recently, threat actors have been identified using QR codes too to embed malicious URLs leading the unsuspecting user to compromised websites containing malware or designed to harvest credentials. In the past month, Darktrace has observed an increase in the number of phishing emails leveraging malicious QR codes for malware distribution and/or credential harvesting, a new form of social engineering attack labelled “Quishing” (i.e., QR code phishing).
Between June 13 and June 22, 2023, Darktrace protected a tech company against one such Quishing attack when five of its senior employees were sent malicious emails impersonating the company’s IT department. The emails contained a QR code that led to a login page designed to harvest the credentials of these senior staff members. Fortunately for the customer, Darktrace / EMAIL thwarted this phishing campaign in the first instance and the emails never reached the employee inboxes.
Trends in Quishing Attacks
The Darktrace/Email team have noticed a recent and rapid increase in QR code abuse, suggesting that it is a growing tactic used by threat actors to deliver malicious payload links. This trend has also been observed by other security solutions [1] [2] [3] [4]. The Darktrace/Email team has identified malicious emails abusing QR codes in multiple ways. Examples include embedded image links which load a QR code and QR code images being delivered as attachments, such as those explored in this case study. Darktrace/Email is continually refining its detection of malicious QR codes and QR code extraction capabilities so that it can detect and block them regardless of their size and location within the email.
Quishing Attack Overview
The attack consisted of five emails, each sent from different sender and envelope addresses, displayed common points between them. The emails all conveyed a sense of urgency, either via the use of words such as “urgent”, “now”, “required” or “important” in the subject field or by marking the email as high priority, thus making the recipient believe the message is pressing and requires immediate attention.
Additionally, the subject of three of the emails directly referred to two factor authentication (2FA) enabling or QR code activation. Another particularity of these emails was that three of them attempted to impersonate the internal IT team of the company by inserting the company domain alongside strings, such as “it-desk” and “IT”, into the personal field of the emails. Email header fields like this are often abused by attackers to trick users by pretending to be an internal department or senior employee, thus avoiding more thorough validation checks. Both instilling a sense of urgency and including a known domain or name in the personal field are techniques that help draw attention to the email and maximize the chances that it is opened and engaged by the recipient.
However, threat actors also need to make sure that the emails actually reach the intended inboxes, and this can be done in several ways. In this case, several tactics were employed. Two of the five emails were sent from legitimate sender addresses that successfully passed SPF validation, suggesting they were sent from compromised accounts. SPF is a standard email authentication method that tells the receiving email servers whether emails have been sent from authorized servers for a given domain. Without SPF validation, emails are more likely to be categorized as spam and be sent to the junk folder as they do not come from authorized sources.
Another of the malicious emails, which also passed SPF checks, used a health care facility company domain in the header-from address field but was actually sent from a different domain (i.e., envelope domain), which lowers the value of the SPF authentication. However, the envelope domain observed in this instance belonged to a company recently acquired by the tech company targeted by the campaign.
This shows a high level of targeting from the attackers, who likely hoped that this detail would make the email more familiar and less suspicious. In another case, the sender domain (i.e., banes-gn[.]com) had been created just 6 days prior, thus lowering the chances of there being open-source intelligence (OSINT) available on the domain. This reduces the chances of the email being detected by traditional email security solutions relying on signatures and known-bad lists.
Darktrace Detects Quishing Attack
Despite its novelty, the domain was detected and assessed as highly suspicious by Darktrace. Darktrace/Email was able to recognize all of the emails as spoofing and impersonation attempts and applied the relevant tags to them, namely “IT Impersonation” and “Fake Account Alert”, depending on the choice of personal field and subject. The senders of the five emails had no prior history or association with the recipient nor the company as no previous correspondence had been observed between the sender and recipient. The tags applied informed on the likely intent and nature of the suspicious indicators present in the email, as shown in Figure 1.

Quishing Attack Tactics
Minimal Plain Text
Another characteristic shared by these emails was that they had little to no text included in the body of the email and they did not contain a plain text portion, as shown in Figure 2. For most normal emails sent by email clients and most automated programs, an email will contain an HTML component and a text component, in addition to any potential attachments present. All the emails had one image attachment, suggesting the bulk of the message was displayed in the image rather than the email body. This hinders textual analysis and filtering of the email for suspicious keywords and language that could reveal its phishing intent. Additionally, the emails were well-formatted and used the logo of the well-known corporation Microsoft, suggesting some level of technical ability on the part of the attackers.

Attachment and link payloads
The threat actors employed some particularly innovative and novel techniques with regards to the attachments and link payloads within these emails. As previously stated, all emails contained an image attachment and one or two links. Figure 3 shows that Darktrace/Email detected that the malicious links present in these emails were located in the attachments, rather than the body of the email. This is a technique often employed by threat actors to bypass link analysis by security gateways. Darktrace/Email was also able to detect this link as a QR code link, as shown in Figure 4.

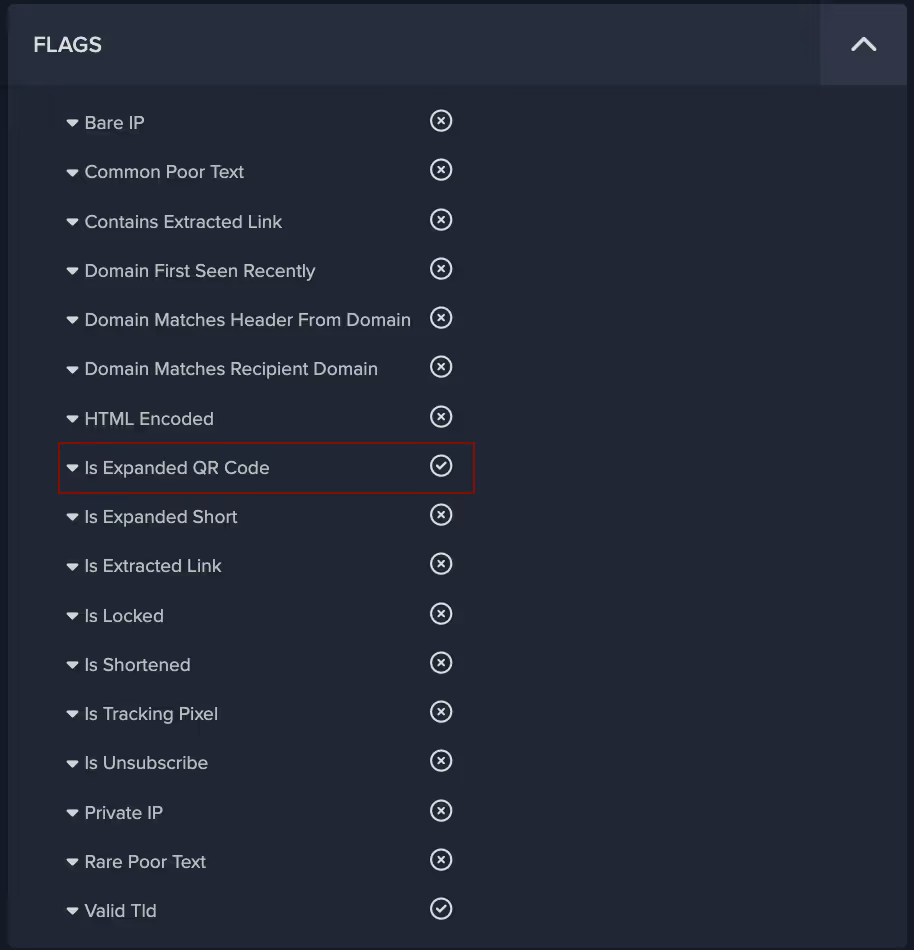
The majority of the text, as well as the malicious payload, was contained within the image attachment, which for one of the emails looked like this:
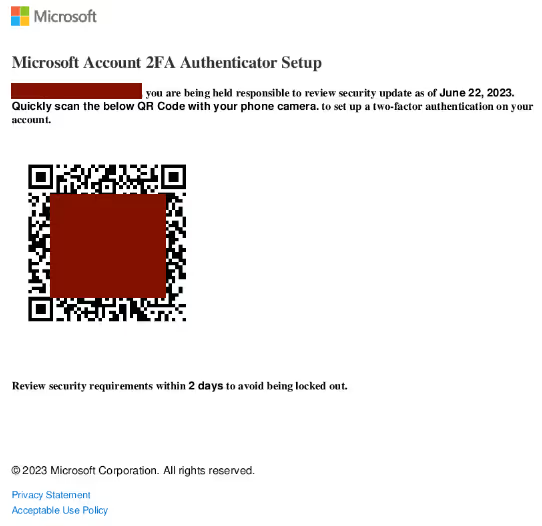
Convincing Appearance
As shown, the recipient is asked to setup 2FA authentication for their account within two days if they don’t want to be locked out. The visual formatting of the image, which includes a corporate logo and Privacy Statement and Acceptable Use Policy notices, is well balanced and convincing. The payload, in this case the QR code containing a malicious link, is positioned in the centre so as to draw attention and encourage the user to scan and click. This is a type of email employees are increasingly accustomed to receiving in order to log into corporate networks and applications. Therefore, recipients of such malicious emails might assume represents expected business activity and thus engage with the QR code without questioning it, especially if the email is claiming to be from the IT department.
Malicious Redirection
Two of the Quishing emails contained links to legitimate file storage and sharing solutions Amazon Web Services (AWS) and and InterPlanetary File System (IPFS), whose domains are less likely to be blocked by traditional security solutions. Additionally, the AWS domain link contained a redirect to a different domain that has been flagged as malicious by multiple security vendors [5]. Malicious redirection was observed in four of the five emails, initially from well-known and benign services’ domains such as bing[.]com and login[.]microsoftonline[.]com. This technique allows attackers to hide the real destination of the link from the user and increase the likelihood that the link is clicked. In two of the emails, the redirect domain had only recently been registered, and in one case, the redirect domain observed was hosted on the new .zip top level domain (i.e., docusafe[.]zip). The domain name suggests it is attempting to masquerade as a compressed file containing important documentation. As seen in Figure 6, a new Darktrace/Email feature allows customers to safely view the final destination of the link, which in this case was a seemingly fake Microsoft login page which could be used to harvest corporate credentials.
.avif)
Gathering Account Credentials
Given the nature of the landing page, it is highly likely that this phishing campaign had the objective of stealing the recipients’ credentials, as further indicated by the presence of the recipients’ email addresses in the links. Additionally, these emails were sent to senior employees, likely in an attempt to gather high value credentials to use in future attacks against the company. Had they succeeded, this would have represented a serious security incident, especially considering that 61% of attacks in 2023 involved stolen or hacked credentials according to Verizon’s 2023 data breach investigations report [6]. However, these emails received the highest possible anomaly score (100%) and were held by Darktrace/Email, thus ensuring that their intended recipients were never exposed to them.
Looking at the indicators of compromise (IoCs) identified in this campaign, it appears that several of the IPs associated with the link payloads have been involved in previous phishing campaigns. Exploring the relations tab for these IPs in Virus Total, some of the communicating files appear to be .eml files and others have generic filenames including strings such as “invoice” “remittance details” “statement” “voice memo”, suggesting they have been involved in other phishing campaigns seemingly related to payment solicitation and other fraud attempts.

Conclusion
Even though the authors of this Quishing campaign used all the tricks in the book to ensure that their emails would arrive unactioned by security tools to the targeted high value recipients’ inboxes, Darktrace/Email was able to immediately recognize the phishing attempts for what they were and block the emails from reaching their destination.
This campaign used both classic and novel tactics, techniques, and procedures, but ultimately were detected and thwarted by Darktrace/Email. It is yet another example of the increasing attack sophistication mentioned in a previous Darktrace blog [7], wherein the attack landscape is moving from low-sophistication, low-impact, and generic phishing tactics to more targeted, sophisticated and higher impact attacks. Darktrace/Email does not rely on historical data nor known-bad lists and is best positioned to protect organizations from these highly targeted and sophisticated attacks.
References
[1] https://www.infosecurity-magazine.com/opinions/qr-codes-vulnerability-cybercrimes/
[2] https://www.helpnetsecurity.com/2023/03/21/qr-scan-scams/
[4] https://businessplus.ie/tech/qr-code-phishing-hp/
[5] https://www.virustotal.com/gui/domain/fistulacure.com
[6] https://www.verizon.com/business/en-gb/resources/reports/dbir/ ; https://www.verizon.com/business/en-gb/resources/reports/dbir/
[7] https://darktrace.com/blog/shifting-email-conversation
Darktrace Model Detections
Association models
No Sender or Content Association
New Sender
Unknown Sender
Low Sender Association
Link models
Focused Link to File Storage
Focused Rare Classified Links
New Unknown Hidden Redirect
High Risk Link + Low Sender Association
Watched Link Type
High Classified Link
File Storage From New
Hidden Link To File Storage
New Correspondent Classified Link
New Unknown Redirect
Rare Hidden Classified Link
Rare Hidden Link
Link To File Storage
Link To File Storage and Unknown Sender
Open Redirect
Unknown Sender Isolated Rare Link
Visually Prominent Link
Visually Prominent Link Unexpected For Sender
Low Link Association
Low Link Association and Unknown Sender
Spoof models
Fake Support Style
External Domain Similarities
Basic Known Entity Similarities
Unusual models
Urgent Request Banner
Urgent Request Banner + Basic Suspicious Sender
Very Young Header Domain
Young Header Domain
Unknown User Tracking
Unrelated Personal Name Address
Unrelated Personal Name Address + Freemail
Unusual Header TLD
Unusual Connection From Unknown
Unbroken Personal
Proximity models
Spam + Unknown Sender
Spam
Spam models
Unlikely Freemail Correspondence
Unlikely Freemail Personalization
General Indicators models
Incoming Mail Security Warning Message
Darktrace Model Tags
Credential Harvesting
Internal IT Impersonation
Multistage payload
Lookalike Domain
Phishing Link
Email Account Takeover
Fake Account Alert
Low Mailing History
No Association
Spoofing Indicators
Unknown Correspondent
VIP
Freemail
IoC - Type - Description & Confidence
fistulacure[.]com
domain
C2 Infrastructure
docusafe[.]zip
domain
Possible C2 Infrastructure
mwmailtec[.]com
domain
Possible C2 Infrastructure
czeromedia[.]com
domain
Possible C2 Infrastructure
192.40.165[.]109
IP address
Probable C2 Infrastructure
209.94.90[.]1
IP address
C2 Infrastructure
52.61.107[.]58
IP address
Possible C2 Infrastructure
40.126.32[.]133
IP address
Possible C2 Infrastructure
211.63.158[.]157
IP address
Possible C2 Infrastructure
119.9.27[.]129
IP address
Possible C2 Infrastructure
184.25.204[.]33
IP address
Possible C2 Infrastructure
40.107.8[.]107
IP address
Probable C2 Infrastructure
40.107.212[.]111
IP address
Possible Infrastructure
27.86.113[.]2
IP address
Possible C2 Infrastructure
192.40.191[.]19
IP address
Possible C2 Infrastructure
157.205.202[.]217
IP address
Possible C2 Infrastructure
a31f1f6063409ecebe8893e36d0048557142cbf13dbaf81af42bf14c43b12a48
SHA256 hash
Possible Malicious File
4c4fb35ab6445bf3749b9d0ab1b04f492f2bc651acb1bbf7af5f0a47502674c9
SHA256 hash
Possible Malicious File
f9c51d270091c34792b17391017a09724d9a7890737e00700dc36babeb97e252
SHA256 hash
Possible Malicious File
9f8ccfd616a8f73c69d25fd348b874d11a036b4d2b3fc7dbb99c1d6fa7413d9a
SHA256 hash
Possible Malicious File
b748894348c32d1dc5702085d70d846c6dd573296e79754df4857921e707c439
SHA256 hash
Possible Malicious File