As-a-Service malware trending
Throughout the course of 2023, “as-a-Service” strains of malware remained the most consistently observed threat type to affect Darktrace customers, mirroring their overall prominence across the cyber threat landscape. With this trend expected to continue throughout 2024, organizations and their security teams should be prepared to defend their network against increasingly versatile and tailorable malware-as-a-service (MaaS) and ransomware-as-a-service (RaaS) strains [1].
What is ALPHV ransomware?
The ALPHV ransomware, also known as ‘BlackCat’ or ‘Noberus’, is one example of a RaaS strain that has been prominent across the threat landscape over the last few years.
ALPHV is a ransomware strain coded in the Rust programming language. The ransomware is sold as part of the RaaS economy [2], with samples of the ransomware being provided and sold by a criminal group (the RaaS ‘operator’) to other cybercriminals (the RaaS ‘affiliates’) who then gain entry to organizations' networks with the intention of detonating the ransomware and demanding ransom payments.
ALPHV was likely first used in the wild back in November 2021 [3]. Since then, it has become one of the most prolific ransomware strains, with the Federal Bureau of Investigation (FBI) reporting nearly USD 300 million in ALPHV ransom payments as of September 2023 [4].
In December 2023, the FBI and the US Department of Justice announced a successful disruption campaign against the ALPHV group, which included a takedown of the their data leak site, and the release of a decryption tool for the ransomware strain [5], and in February 2024, the US Department of State announced a reward of up to USD 10 million for information leading to the identification or location of anyone occupying a key leadership position in the group operating the ALPHV ransomware strain [6].
The disruption campaign against the ransomware group appeared to have been successful, as evidenced by the recent, significant decline in ALPHV attacks, however, it would not be surprising for the group to simply return with new branding, in a similar vein to its apparent predecessors, DarkSide and BlackMatter [7].
How does ALPHV ransomware work?
ALPHV affiliates have been known to employ a variety of methods to progress towards their objective of detonating ALPHV ransomware [4]. In the latter half of 2023, ALPHV affiliates were observed using malicious advertising (i.e, malvertising) to deliver a Python-based backdoor-dropper known as 'Nitrogen' to users' devices [8][12]. These malvertising operations consisted in affiliates setting up malicious search engine adverts for tools such as WinSCP and AnyDesk.
Users' interactions with these adverts led them to sites resembling legitimate software distribution sites. Users' attempts to download software from these spoofed sites resulted in the delivery of a backdoor-dropping malware sample dubbed 'Nitrogen' to their devices. Nitrogen has been observed dropping a variety of command-and-control (C2) implants onto users' devices, including Cobalt Strike Beacon and Sliver C2. ALPHV affiliates often used the backdoor access afforded to them by these C2 implants to conduct reconnaissance and move laterally, in preparation for detonating ALPHV ransomware payloads.
Darktrace Detection of ALPHV Ransomware
During October 2023, Darktrace observed several cases of ALPHV affiliates attempting to infiltrate organizations' networks via the use of malvertising to socially engineer users into downloading and installing Nitrogen from impersonation websites such as 'wireshhark[.]com' and wìnscp[.]net (i.e, xn--wnscp-tsa[.]net).
While the attackers managed to bypass traditional security measures and evade detection by using a device from the customer’s IT team to perform its malicious activity, Darktrace DETECT™ swiftly identified the subtle indicators of compromise (IoCs) in the first instance. This swift detection of ALPHV, along with Cyber AI Analyst™ autonomously investigating the wide array of post-compromise activity, provided the customer with full visibility over the attack enabling them to promptly initiate their remediation and recovery efforts.
Unfortunately, in this incident, Darktrace RESPOND™ was not fully deployed within their environment, hindering its ability to autonomously counter emerging threats. Had RESPOND been fully operational here, it would have effectively contained the attack in its early stages, avoiding the eventual detonation of the ALPHV ransomware.
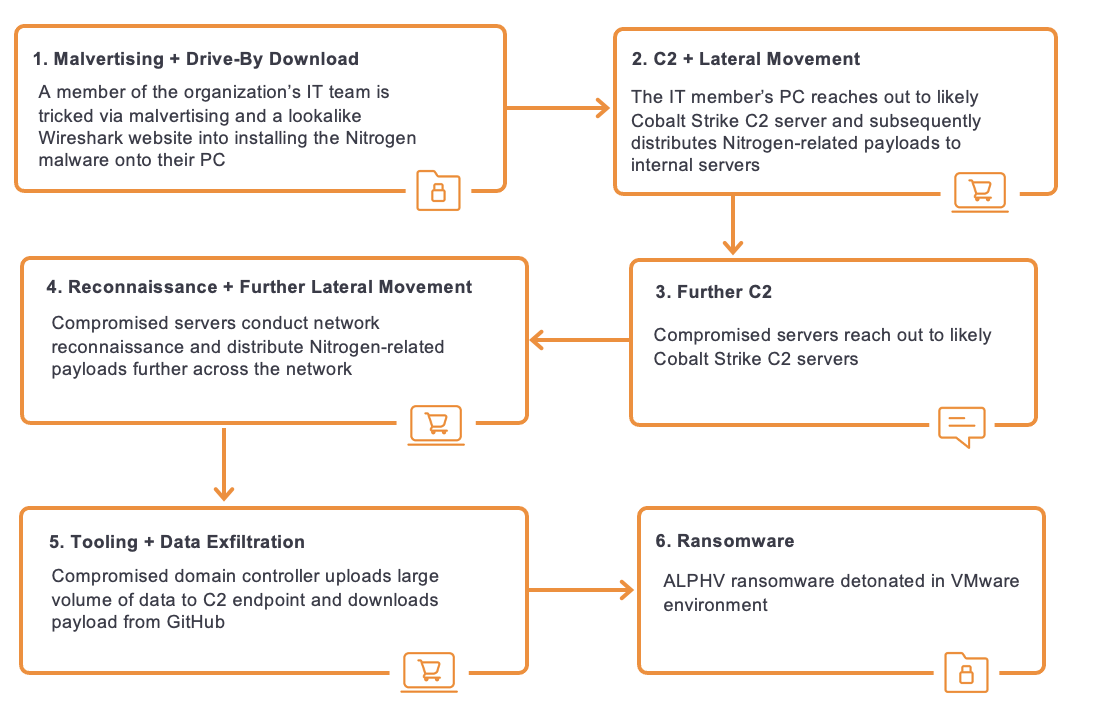
In mid-October, a member of the IT team at a US-based Darktrace customer attempted to install the network traffic analysis software, Wireshark, onto their desktop. Due to the customer’s configuration, Darktrace's visibility over this device was limited to its internal traffic, despite this it was still able to identify and alert for a string of suspicious activity conducted by the device.
Initially, Darktrace observed the device making type A DNS requests for 'wiki.wireshark[.]org' immediately before making type A DNS requests for the domain names 'www.googleadservices[.]com', 'allpcsoftware[.]com', and 'wireshhark[.]com' (note the two 'h's). This pattern of activity indicates that the device’s user was redirected to the website, wireshhark[.]com, as a result of the user's interaction with a sponsored Google Search result pointing to allpcsoftware[.]com.
At the time of analysis, navigating to wireshhark[.]com directly from the browser search bar led to a YouTube video of Rick Astley's song "Never Gonna Give You Up". This suggests that the website, wireshhark[.]com, had been configured to redirect users to this video unless they had arrived at the website via the relevant sponsored Google Search result [8].
Although it was not possible to confirm this with certainty, it is highly likely that users who visited the website via the appropriate sponsored Google Search result were led to a fake website (wireshhark[.]com) posing as the legitimate website, wireshark[.]com. It seems that the actors who set up this fake version of wireshark[.]com were inspired by the well-known bait-and-switch technique known as 'rickrolling', where users are presented with a desirable lure (typically a hyperlink of some kind) which unexpectedly leads them to a music video of Rick Astley's "Never Gonna Give You Up".
After being redirected to wireshhark[.]com, the user unintentionally installed a malware sample which dropped what appears to be Cobalt Strike onto their device. The presence of Cobalt Strike on the user's desktop was evidenced by the subsequent type A DNS requests which the device made for the domain name 'pse[.]ac'. These DNS requests were responded to with the likely Cobalt Strike C2 server address, 194.169.175[.]132. Given that Darktrace only had visibility over the device’s internal traffic, it did not observe any C2 connections to this Cobalt Strike endpoint. However, the desktop's subsequent behavior suggests that a malicious actor had gained 'hands-on-keyboard' control of the device via an established C2 channel.
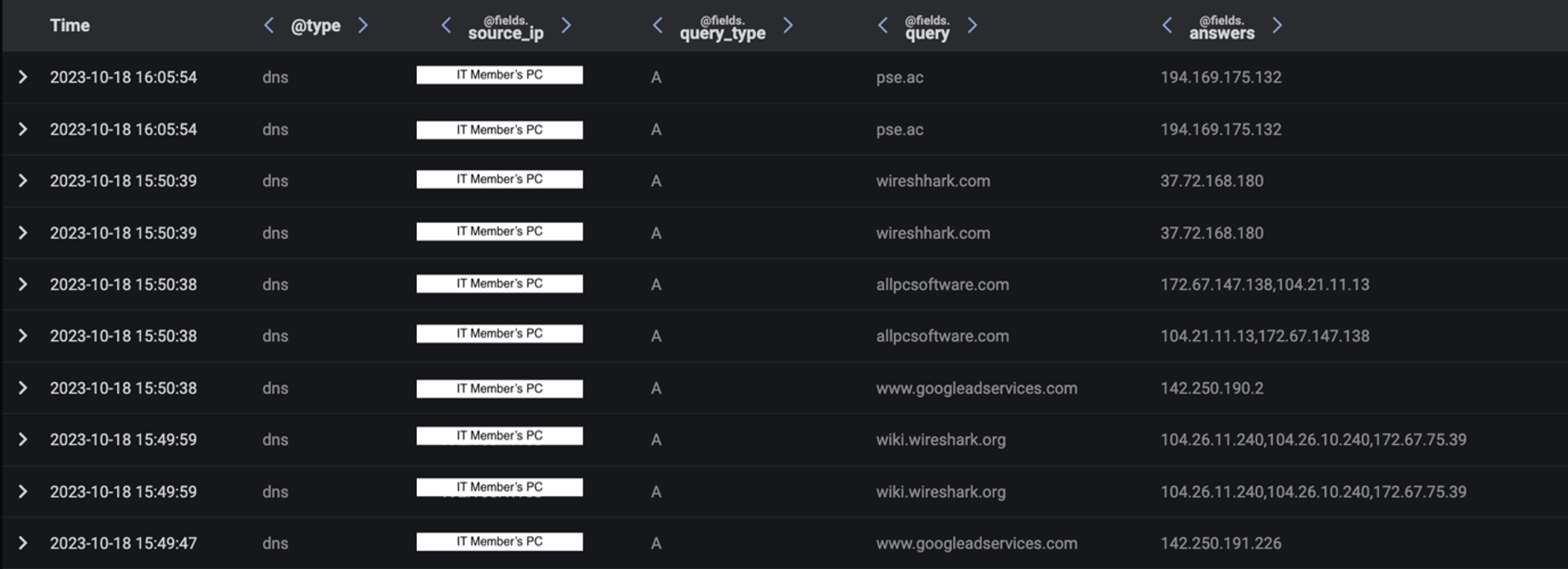
Since the malicious actor had gained control of an IT member's device, they were able to abuse the privileged account credentials to spread Python payloads across the network via SMB and the Windows Management Instrumentation (WMI) service. The actor was also seen distributing the Windows Sys-Internals tool, PsExec, likely in an attempt to facilitate their lateral movement efforts. It was normal for this IT member's desktop to distribute files across the network via SMB, which meant that this malicious SMB activity was not, at first glance, out of place.
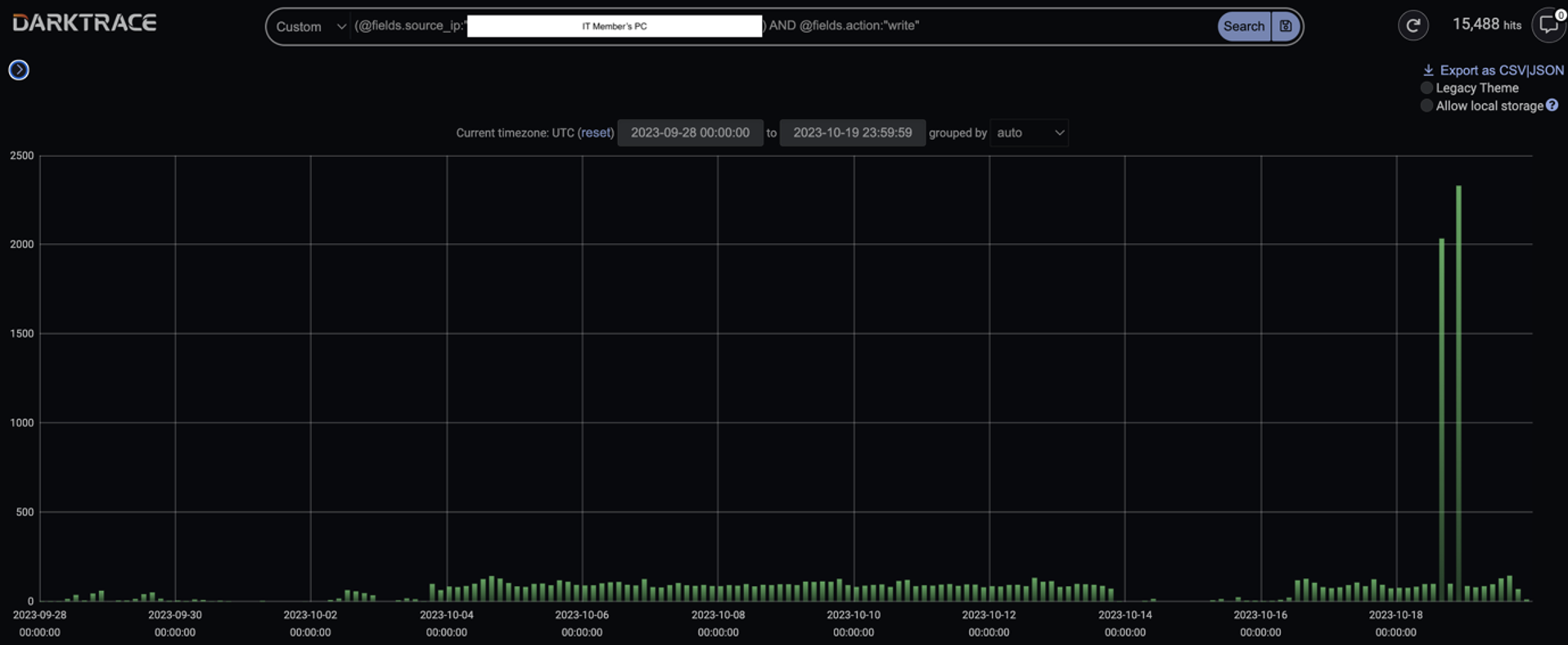
However, Darktrace DETECT recognized that the significant spike in file writes being performed here was suspicious, even though, on the surface, it seemed ‘normal’ for the device. Furthermore, Darktrace identified that the executable files being distributed were attempting to masquerade as a different file type, potentially in an attempt to evade the detection of traditional security tools.
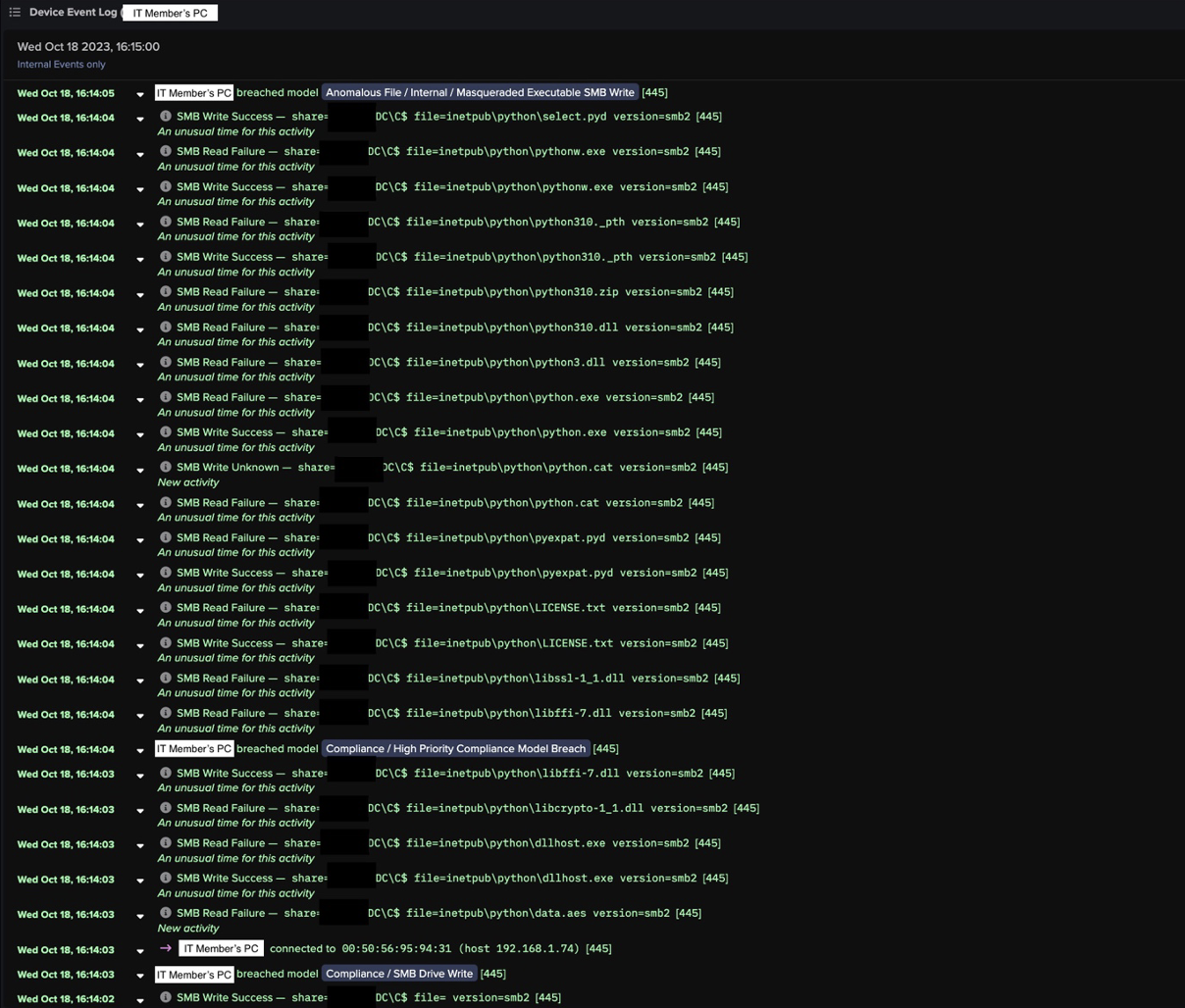
An addition to DETECT’s identification of this unusual activity, Darktrace’s Cyber AI Analyst launched an autonomous investigation into the ongoing compromise and was able to link the SMB writes and the sharing of the executable Python payloads, viewing the connections as one lateral movement incident rather than a string of isolated events. After completing its investigation, Cyber AI Analyst was able to provide a detailed summary of events on one pane of glass, ensuring the customer could identify the affected device and begin their remediation.
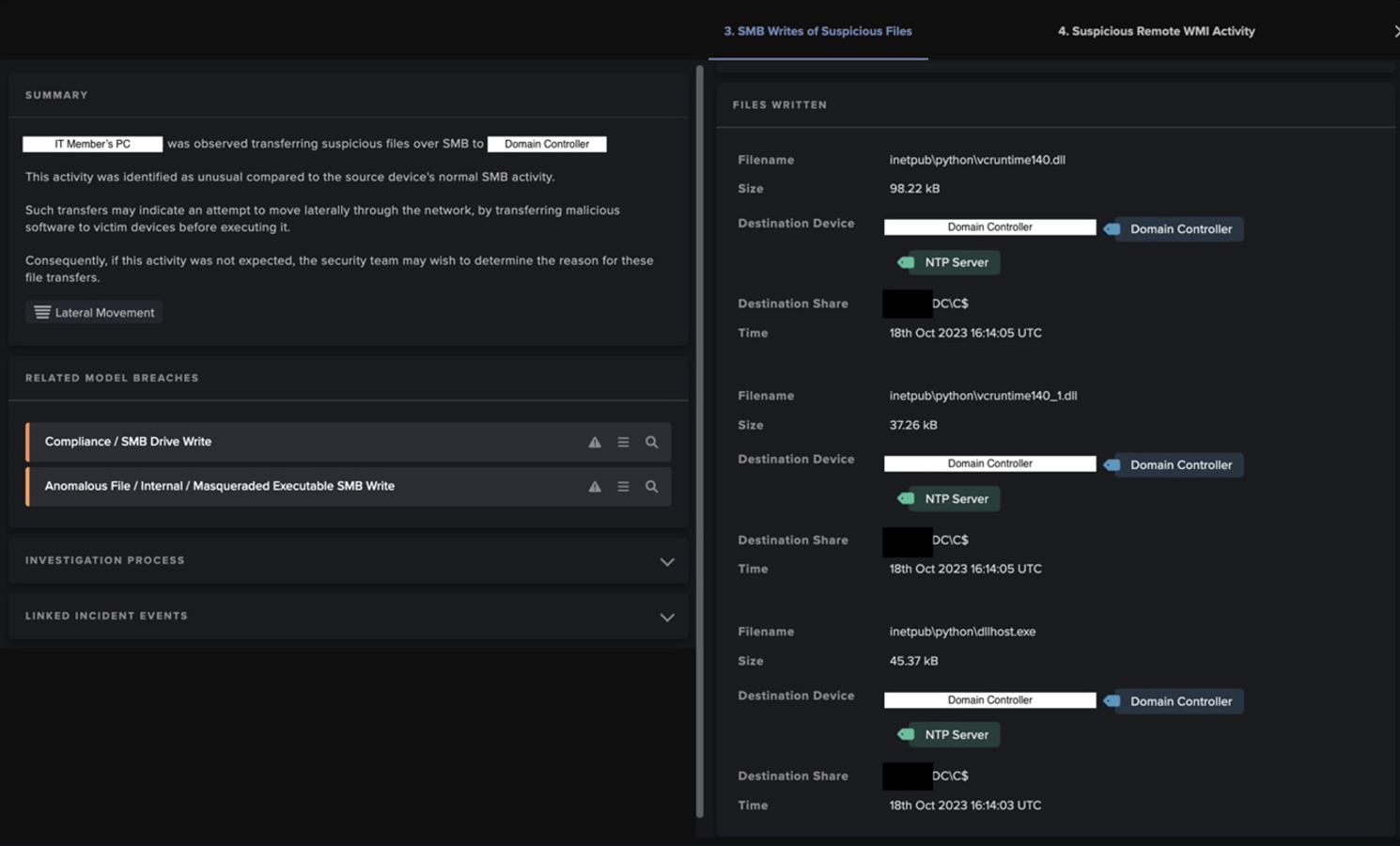
C2 Activity
The Python payloads distributed by the IT member’s device were likely related to the Nitrogen malware, as evidenced by the payloads’ names and by the network behaviours which they engendered.
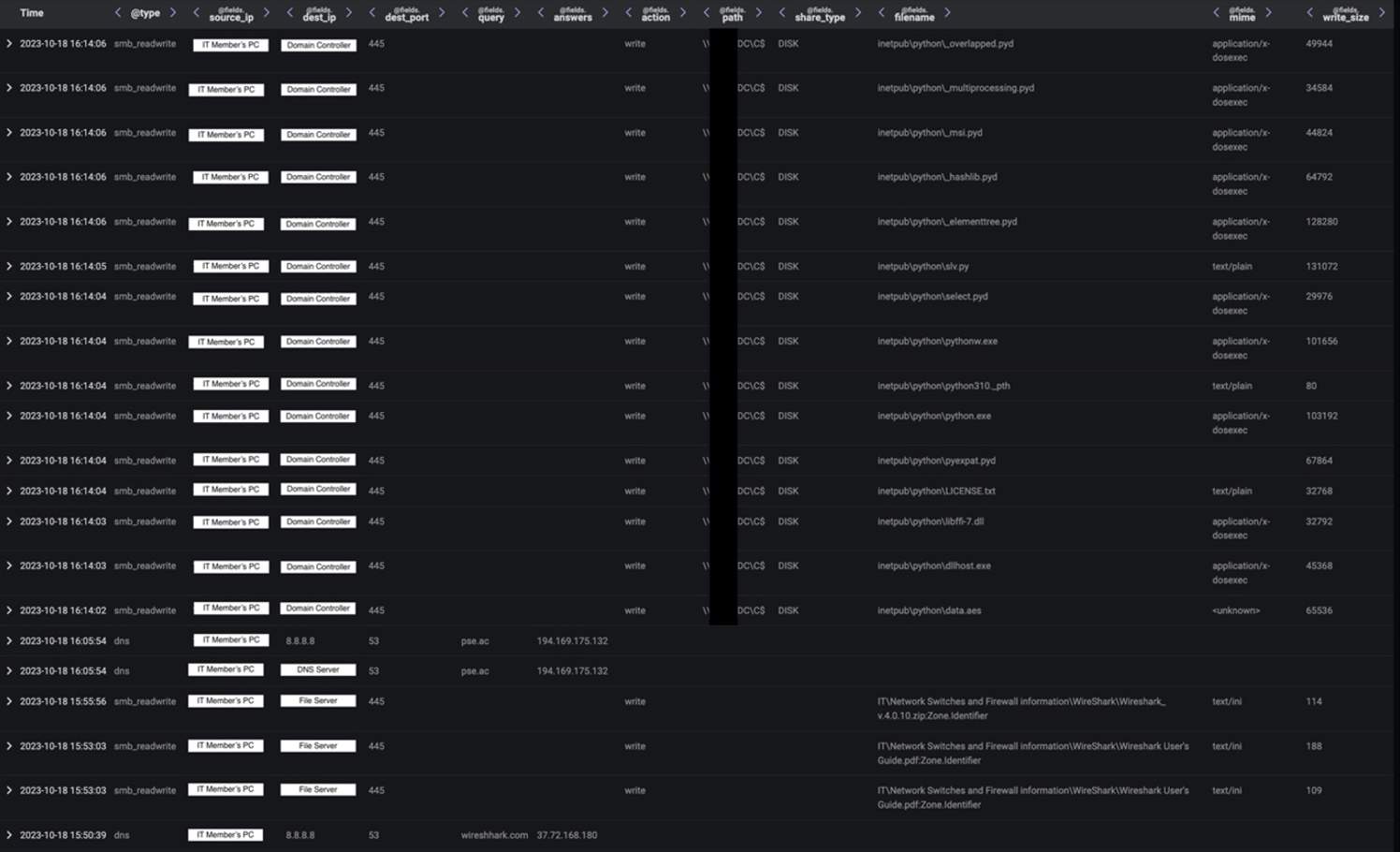
The internal devices to which these Nitrogen payloads were distributed immediately went on to contact C2 infrastructure associated with Cobalt Strike. These C2 connections were made over SSL on ports 443 and 8443. Darktrace identified the attacker moving laterally to an internal SQL server and an internal domain controller.
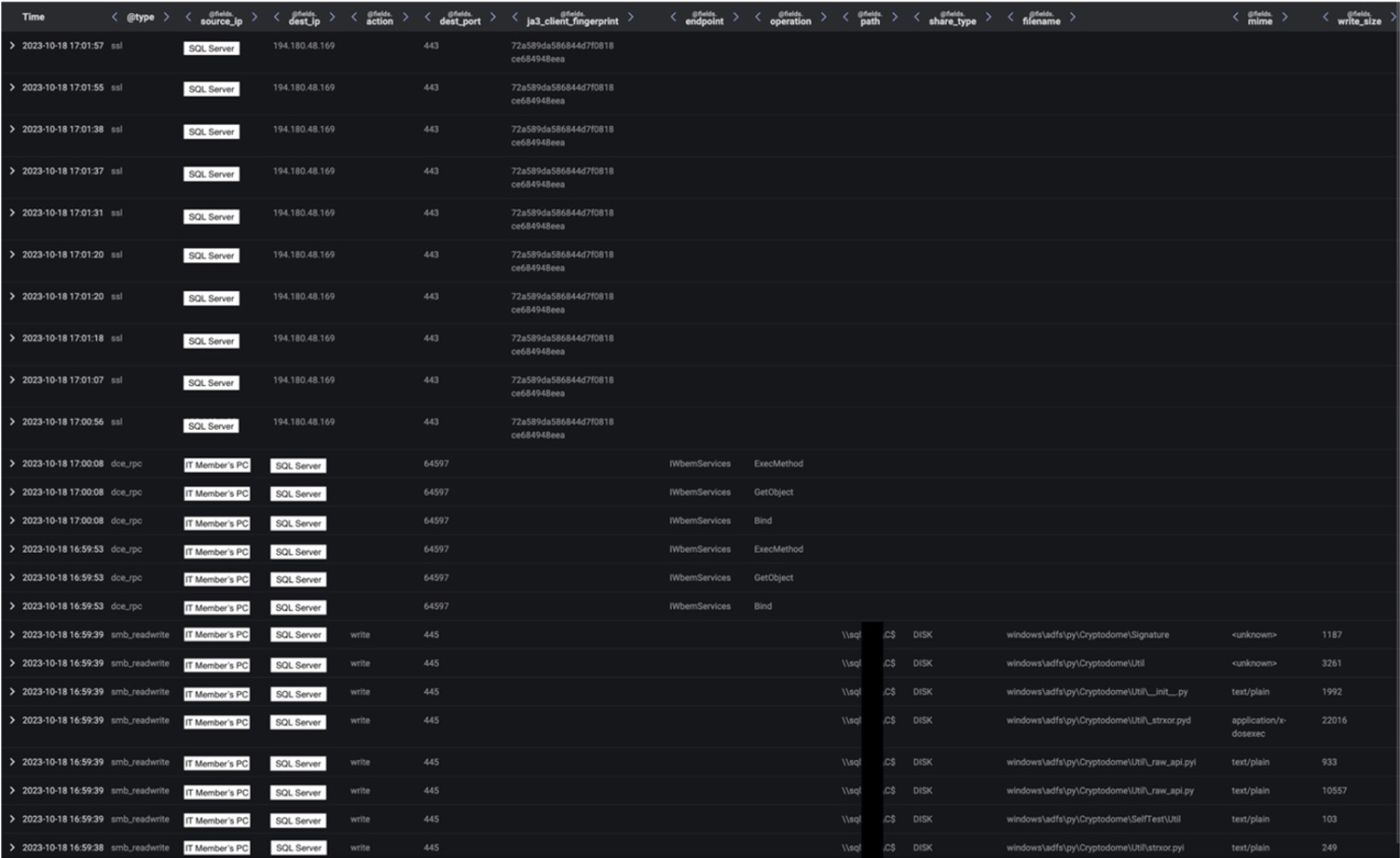
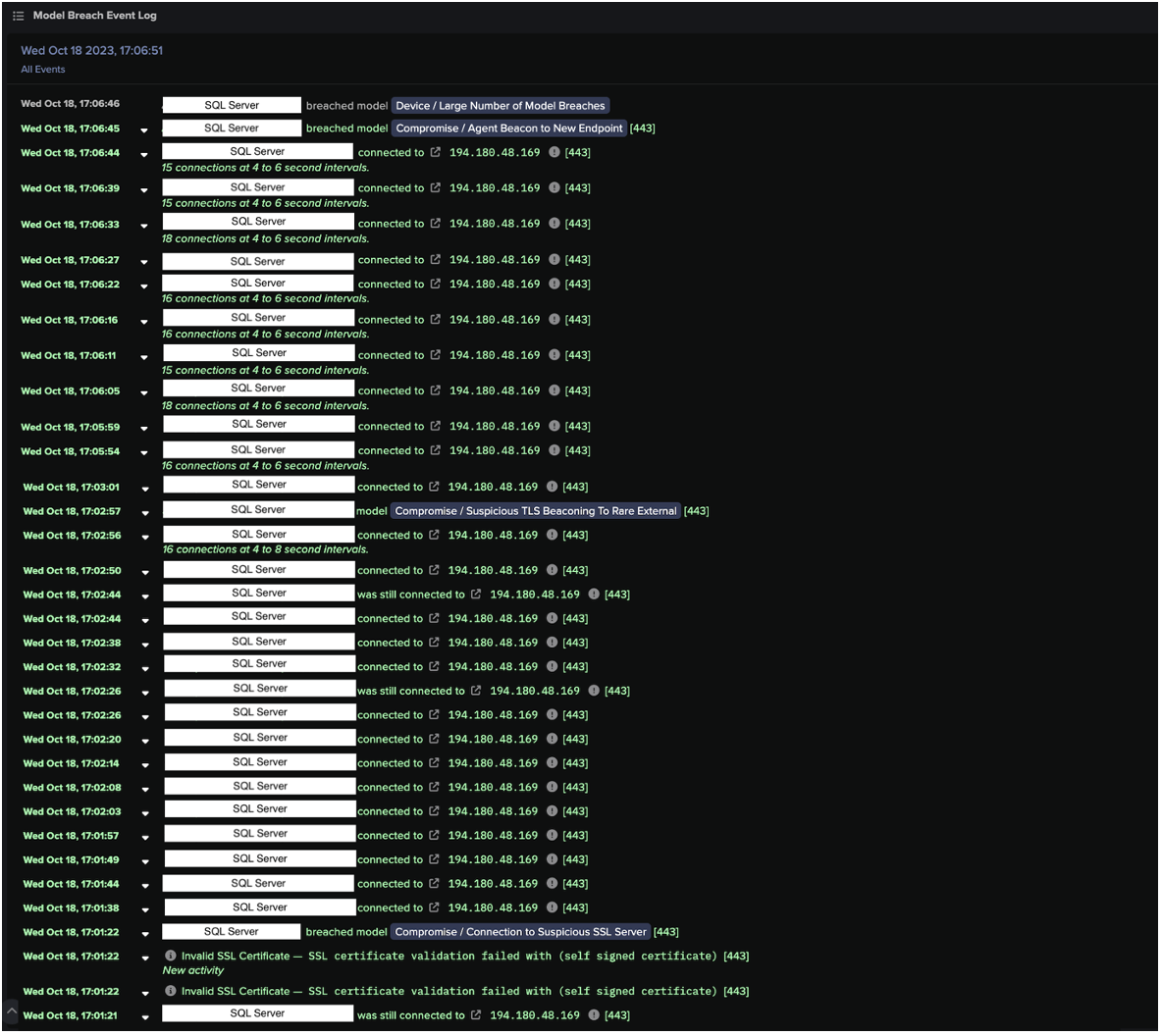
Once more, Cyber AI Analyst launched its own investigation into this activity and was able to successfully identify a series of separate SSL connections, linking them together into one wider C2 incident.
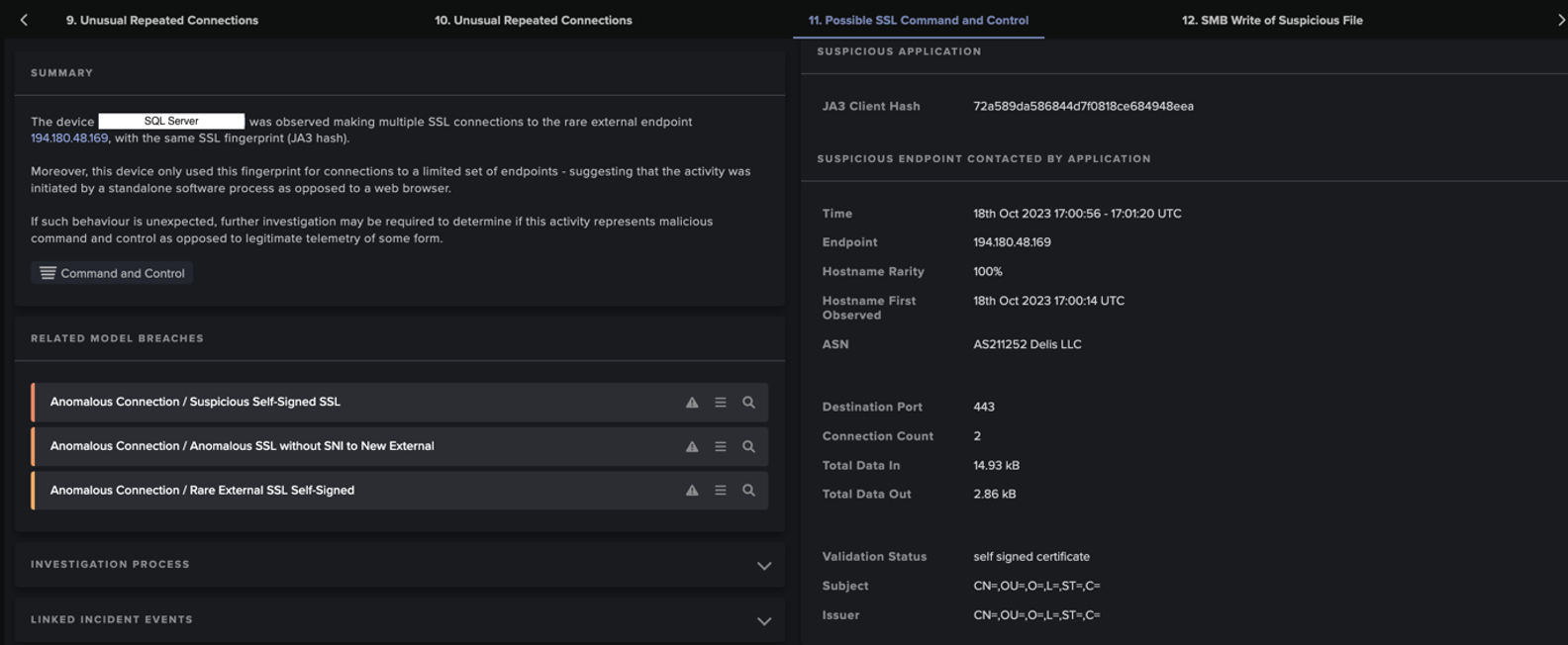
Darktrace observed the attacker using their 'hands-on-keyboard' access to these systems to elevate their privileges, conduct network reconnaissance (primarily port scanning), spread Python payloads further across the network, exfiltrate data from the domain controller and transfer a payload from GitHub to the domain controller.
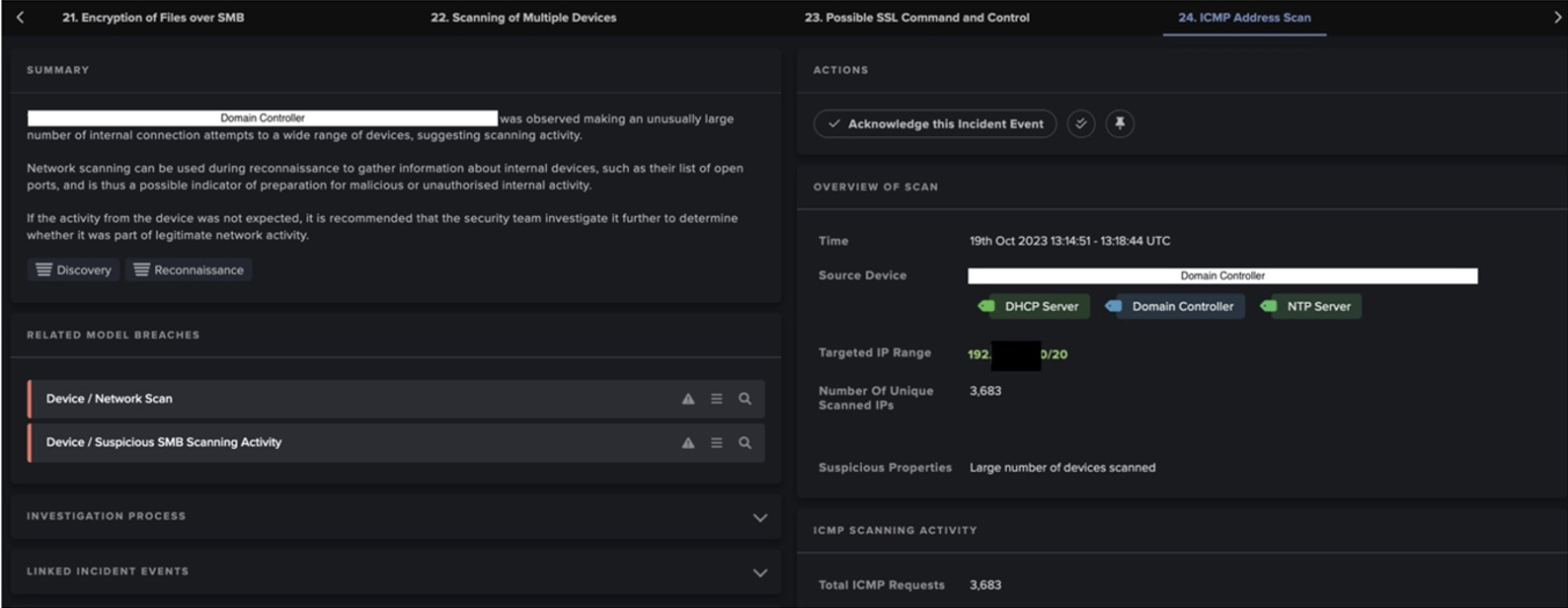

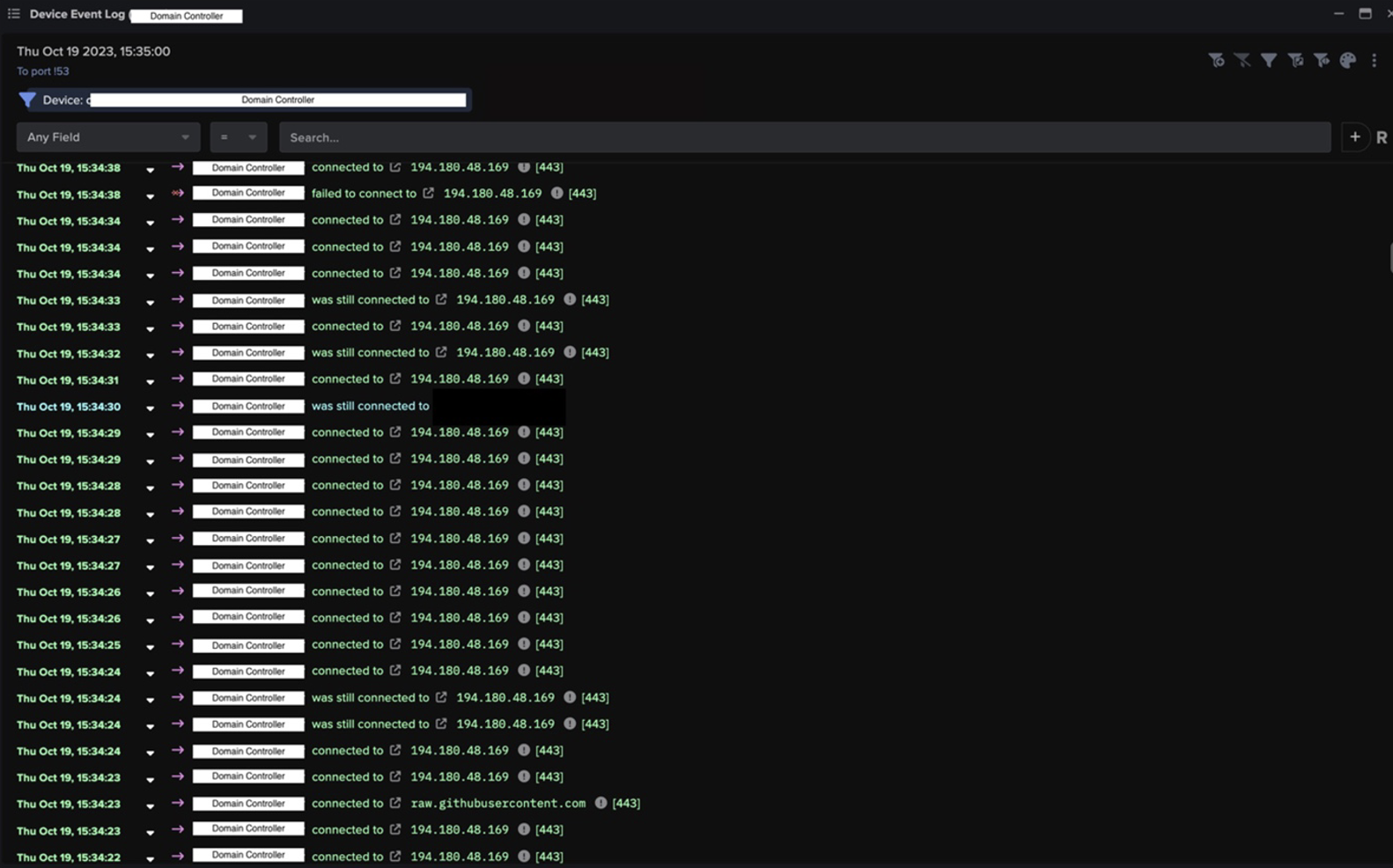
After conducting extensive reconnaissance and lateral movement activities, the attacker was observed detonating ransomware with the organization's VMware environment, resulting in the successful encryption of the customer’s VMware vCenter server and VMware virtual machines. In this case, the attacker took around 24 hours to progress from initial access to ransomware detonation.
If the targeted organization had been signed up for Darktrace's Proactive Threat Notification (PTN) service, they would have been promptly notified of these suspicious activities by the Darktrace Security Operations Center (SOC) in the first instance, allowing them to quickly identify affected devices and quarantine them before the compromise could escalate.
Additionally, given the quantity of high-severe alerts that triggered in response to this attack, Darktrace RESPOND would, under normal circumstances, have inhibited the attacker's activities as soon as they were identified by DETECT. However, due to RESPOND not being configured to act on server devices within the customer’s network, the attacker was able to seamlessly move laterally through the organization's server environment and eventually detonate the ALPHV ransomware.
Nevertheless, Darktrace was able to successfully weave together multiple Cyber AI Analyst incidents which it generated into a thread representing the chain of behavior that made up this attack. The thread of Incident Events created by Cyber AI Analyst provided a substantial account of the attack and the steps involved in it, which significantly facilitated the customer’s post-incident investigation efforts.
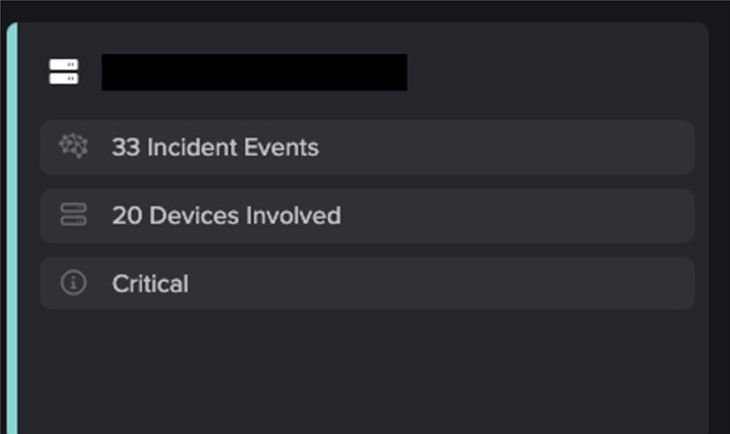
Conclusion
It is expected for malicious cyber actors to revise and upgrade their methods to evade organizations’ improving security measures. The continued improvement of email security tools, for example, has likely created a need for attackers to develop new means of Initial Access, such as the use of Microsoft Teams-based malware delivery.
This fast-paced ALPHV ransomware attack serves as a further illustration of this trend, with the actor behind the attack using malvertising to convince an unsuspecting user to download the Python-based malware, Nitrogen, from a fake Wireshark site. Unbeknownst to the user, this stealthy malware dropped a C2 implant onto the user’s device, giving the malicious actor the ‘hands-on-keyboard’ access they needed to move laterally, conduct network reconnaissance, and ultimately detonate ALPHV ransomware.
Despite the non-traditional initial access methods used by this ransomware actor, Darktrace DETECT was still able to identify the unusual patterns of network traffic caused by the attacker’s post-compromise activities. The large volume of alerts created by Darktrace DETECT were autonomously investigated by Darktrace’s Cyber AI Analyst, which was able to weave together related activities of different devices into a comprehensive timeline of the attacker’s operation. Given the volume of DETECT alerts created in response to this ALPHV attack, it is expected that Darktrace RESPOND would have autonomously inhibited the attacker’s operation had the capability been appropriately configured.
As the first post-compromise activities Darktrace observed in this ALPHV attack were seemingly performed by a member of the customer’s IT team, it may have looked normal to a human or traditional signature and rules-based security tools. To Darktrace’s Self-Learning AI, however, the observed activities represented subtle deviations from the device’s normal pattern of life. This attack, and Darktrace’s detection of it, is therefore a prime illustration of the value that Self-Learning AI can bring to the task of detecting anomalies within organizations’ digital estates.
Credit to Sam Lister, Senior Cyber Analyst, Emma Foulger, Principal Cyber Analyst
Appendices
Darktrace DETECT Model Breaches
- Compliance / SMB Drive Write
- Compliance / High Priority Compliance Model Breach
- Anomalous File / Internal / Masqueraded Executable SMB Write
- Device / New or Uncommon WMI Activity
- Anomalous Connection / New or Uncommon Service Control
- Anomalous Connection / High Volume of New or Uncommon Service Control
- Device / New or Uncommon SMB Named Pipe
- Device / Multiple Lateral Movement Model Breaches
- Device / Large Number of Model Breaches
- SMB Writes of Suspicious Files (Cyber AI Analyst)
- Suspicious Remote WMI Activity (Cyber AI Analyst)
- Suspicious DCE-RPC Activity (Cyber AI Analyst)
- Compromise / Connection to Suspicious SSL Server
- Compromise / High Volume of Connections with Beacon Score
- Anomalous Connection / Suspicious Self-Signed SSL
- Anomalous Connection / Anomalous SSL without SNI to New External
- Compromise / Suspicious TLS Beaconing To Rare External
- Compromise / Beacon to Young Endpoint
- Compromise / SSL or HTTP Beacon
- Compromise / Agent Beacon to New Endpoint
- Device / Long Agent Connection to New Endpoint
- Compromise / SSL Beaconing to Rare Destination
- Compromise / Large Number of Suspicious Successful Connections
- Compromise / Slow Beaconing Activity To External Rare
- Anomalous Server Activity / Outgoing from Server
- Device / Multiple C2 Model Breaches
- Possible SSL Command and Control (Cyber AI Analyst)
- Unusual Repeated Connections (Cyber AI Analyst)
- Device / ICMP Address Scan
- Device / RDP Scan
- Device / Network Scan
- Device / Suspicious Network Scan Activity
- Scanning of Multiple Devices (Cyber AI Analyst)
- ICMP Address Scan (Cyber AI Analyst)
- Device / Anomalous Github Download
- Unusual Activity / Unusual External Data Transfer
- Device / Initial Breach Chain Compromise
MITRE ATT&CK Mapping
Resource Development techniques:
- Acquire Infrastructure: Malvertising (T1583.008)
Initial Access techniques:
- Drive-by Compromise (T1189)
Execution techniques:
- User Execution: Malicious File (T1204.002)
- System Services: Service Execution (T1569.002)
- Windows Management Instrumentation (T1047)
Defence Evasion techniques:
- Masquerading: Match Legitimate Name or Location (T1036.005)
Discovery techniques:
- Remote System Discovery (T1018)
- Network Service Discovery (T1046)
Lateral Movement techniques:
- Remote Services: SMB/Windows Admin Shares
- Lateral Tool Transfer (T1570)
Command and Control techniques:
- Application Layer Protocol: Web Protocols (T1071.001)
- Encrypted Channel: Asymmetric Cryptography (T1573.002)
- Non-Standard Port (T1571)
- Ingress Tool Channel (T1105)
Exfiltration techniques:
- Exfiltration Over C2 Channel (T1041)
Impact techniques:
- Data Encrypted for Impact (T1486)
List of Indicators of Compromise
- allpcsoftware[.]com
- wireshhark[.]com
- pse[.]ac • 194.169.175[.]132
- 194.180.48[.]169
- 193.42.33[.]14
- 141.98.6[.]195
References
[1] https://darktrace.com/threat-report-2023
[2] https://www.microsoft.com/en-us/security/blog/2022/05/09/ransomware-as-a-service-understanding-the-cybercrime-gig-economy-and-how-to-protect-yourself/
[4] https://www.cisa.gov/news-events/cybersecurity-advisories/aa23-353a
[5] https://www.justice.gov/opa/pr/justice-department-disrupts-prolific-alphvblackcat-ransomware-variant
[6] https://www.state.gov/u-s-department-of-state-announces-reward-offers-for-criminal-associates-of-the-alphv-blackcat-ransomware-variant/
[7] https://www.bleepingcomputer.com/news/security/blackcat-alphv-ransomware-linked-to-blackmatter-darkside-gangs/
[8] https://www.trendmicro.com/en_us/research/23/f/malvertising-used-as-entry-vector-for-blackcat-actors-also-lever.html
[9] https://news.sophos.com/en-us/2023/07/26/into-the-tank-with-nitrogen/
[10] https://www.esentire.com/blog/persistent-connection-established-nitrogen-campaign-leverages-dll-side-loading-technique-for-c2-communication
[11] https://www.esentire.com/blog/nitrogen-campaign-2-0-reloads-with-enhanced-capabilities-leading-to-alphv-blackcat-ransomware
[12] https://www.esentire.com/blog/the-notorious-alphv-blackcat-ransomware-gang-is-attacking-corporations-and-public-entities-using-google-ads-laced-with-malware-warns-esentire