Trending blogs
Inside the SOC
A series exclusively authored by Darktrace's expert cyber analysts, containing technical deep dives of cyber incidents and the latest threat trends.
Thank you! Your submission has been received!
No results found
Please try using a different search or filter
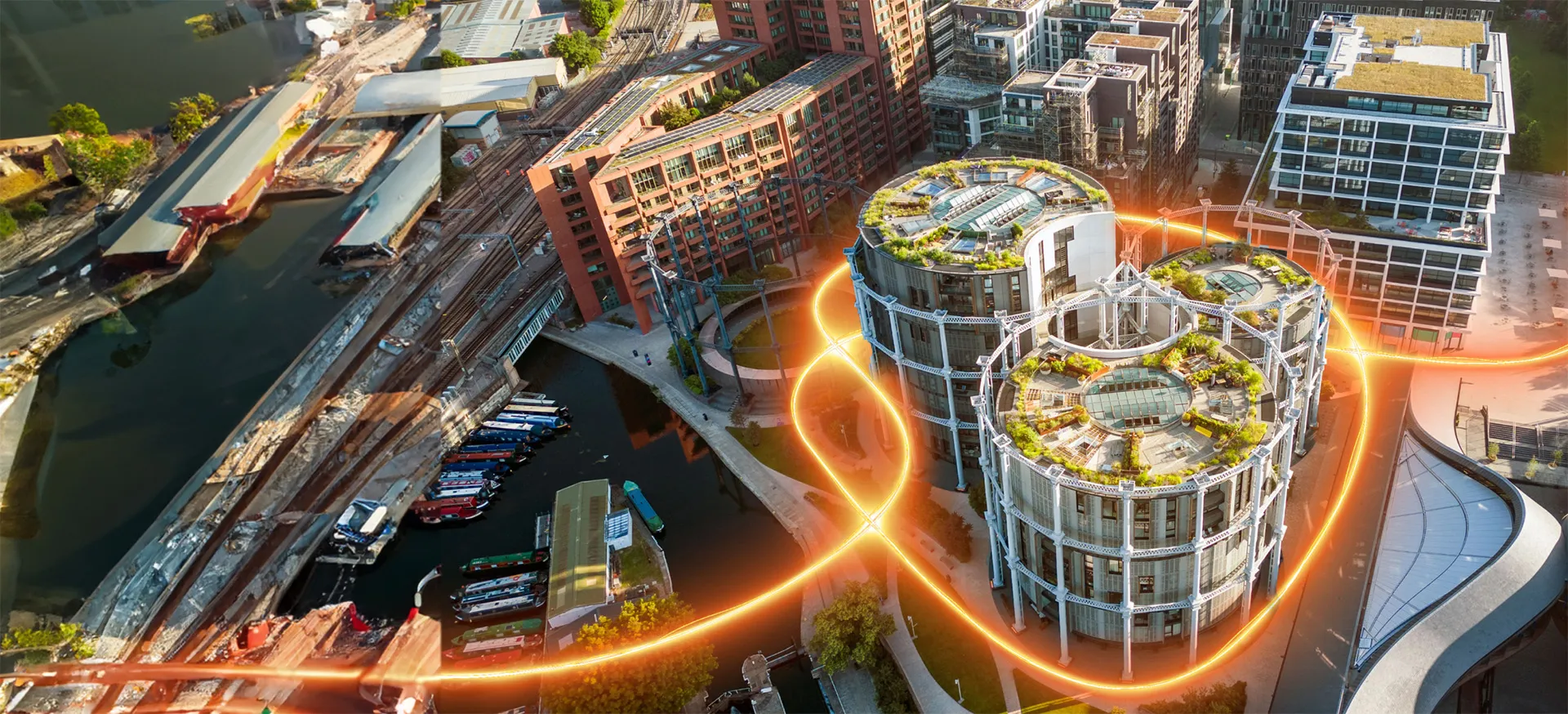